One of the most important and interesting topics under Linux administration is I/O redirection. This feature of the command line enables you to redirect the input and/or output of commands from and/or to files, or join multiple commands together using pipes to form what is known as a “command pipeline”.
All the commands that we run fundamentally produce two kinds of output:
- the command result – data the program is designed to produce, and
- the program status and error messages that informs a user of the program execution details.
In Linux and other Unix-like systems, there are three default files named below which are also identified by the shell using file descriptor numbers:
- stdin or 0 – it’s connected to the keyboard, most programs read input from this file.
- stdout or 1 – it’s attached to the screen, and all programs send their results to this file and
- stderr or 2 – programs send status/error messages to this file which is also attached to the screen.
Therefore, I/O redirection allows you to alter the input source of a command as well as where its output and error messages are sent to. And this is made possible by the “<”
and “>”
redirection operators.
How To Redirect Standard Output to File in Linux
You can redirect standard output as in the example below, here, we want to store the output of the top command for later inspection:
$ top -bn 5 >top.log
Where the flags:
-b
– enables top to run in batch mode, so that you can redirect its output to a file or another command.-n
– specifies the number of iterations before the command terminates.
You can view the contents of top.log
file using cat command as follows:
$ cat top.log
To append the output of a command, use the “>>”
operator.
For instance to append the output of top command above in the top.log file especially within a script (or on the command line), enter the line below:
$ top -bn 5 >>top.log
Note: Using the file descriptor number, the output redirect command above is the same as:
$ top -bn 5 1>top.log
How To Redirect Standard Error to File in Linux
To redirect standard error of a command, you need to explicitly specify the file descriptor number, 2
for the shell to understand what you are trying to do.
For example the ls command below will produce an error when executed by a normal system user without root privileges:
$ ls -l /root/
You can redirect the standard error to a file as below:
$ ls -l /root/ 2>ls-error.log $ cat ls-error.log
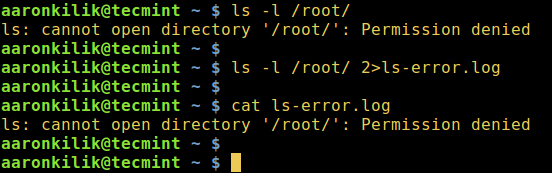
In order to append the standard error, use the command below:
$ ls -l /root/ 2>>ls-error.log
How To Redirect Standard Output/ Error To One File
It is also possible to capture all the output of a command (both standard output and standard error) into a single file. This can be done in two possible ways by specifying the file descriptor numbers:
1. The first is a relatively old method which works as follows:
$ ls -l /root/ >ls-error.log 2>&1
The command above means the shell will first send the output of the ls command to the file ls-error.log (using >ls-error.log
), and then writes all error messages to the file descriptor 2 (standard output) which has been redirected to the file ls-error.log (using 2>&1
). Implying that standard error is also sent to the same file as standard output.
2. The second and direct method is:
$ ls -l /root/ &>ls-error.log
You can as well append standard output and standard error to a single file like so:
$ ls -l /root/ &>>ls-error.log
How To Redirect Standard Input to File
Most if not all commands get their input from standard input, and by default standard input is attached to the keyboard.
To redirect standard input from a file other than the keyboard, use the “<”
operator as below:
$ cat <domains.list
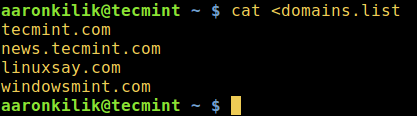
How To Redirect Standard Input/Output to File
You can perform standard input, standard output redirection at the same time using sort command as below:
$ sort <domains.list >sort.output
How to Use I/O Redirection Using Pipes
To redirect the output of one command as input of another, you can use pipes, this is a powerful means of building useful command lines for complex operations.
For example, the command below will list the top five recently modified files.
$ ls -lt | head -n 5
Here, the options:
-l
– enables long listing format-t
– sort by modification time with the newest files are shown first-n
– specifies the number of header lines to show
Important Commands for Building Pipelines
Here, we will briefly review two important commands for building command pipelines and they are:
xargs which is used to build and execute command lines from standard input. Below is an example of a pipeline which uses xargs, this command is used to copy a file into multiple directories in Linux:
$ echo /home/aaronkilik/test/ /home/aaronkilik/tmp | xargs -n 1 cp -v /home/aaronkilik/bin/sys_info.sh

And the options:
-n 1
– instructs xargs to use at most one argument per command line and send to the cp commandcp
– copies the file-v
– displays progress of copy command.
For more usage options and info, read through the xargs man page:
$ man xargs
A tee command reads from standard input and writes to standard output and files. We can demonstrate how tee works as follows:
$ echo "Testing how tee command works" | tee file1

File or text filters are commonly used with pipes for effective Linux file operations, to process information in powerful ways such as restructuring output of commands (this can be vital for generation of useful Linux reports), modifying text in files plus several other Linux system administration tasks.
To learn more about Linux filters and pipes, read this article Find Top 10 IP Addresses Accessing Apache Server, shows a useful example of using filters and pipes.
In this article, we explained the fundamentals of I/O redirection in Linux. Remember to share your thoughts via the feedback section below.
You didn’t cover the ‘here doc’ redirecting input from within the script file using << terminator … terminator
sendmail [email protected] [email protected] <<****
This is a test message
with ${SUBSTITUTE} of text
.
****
@Richard
Many thanks for the reminder, we will update article to include ‘here doc’.